
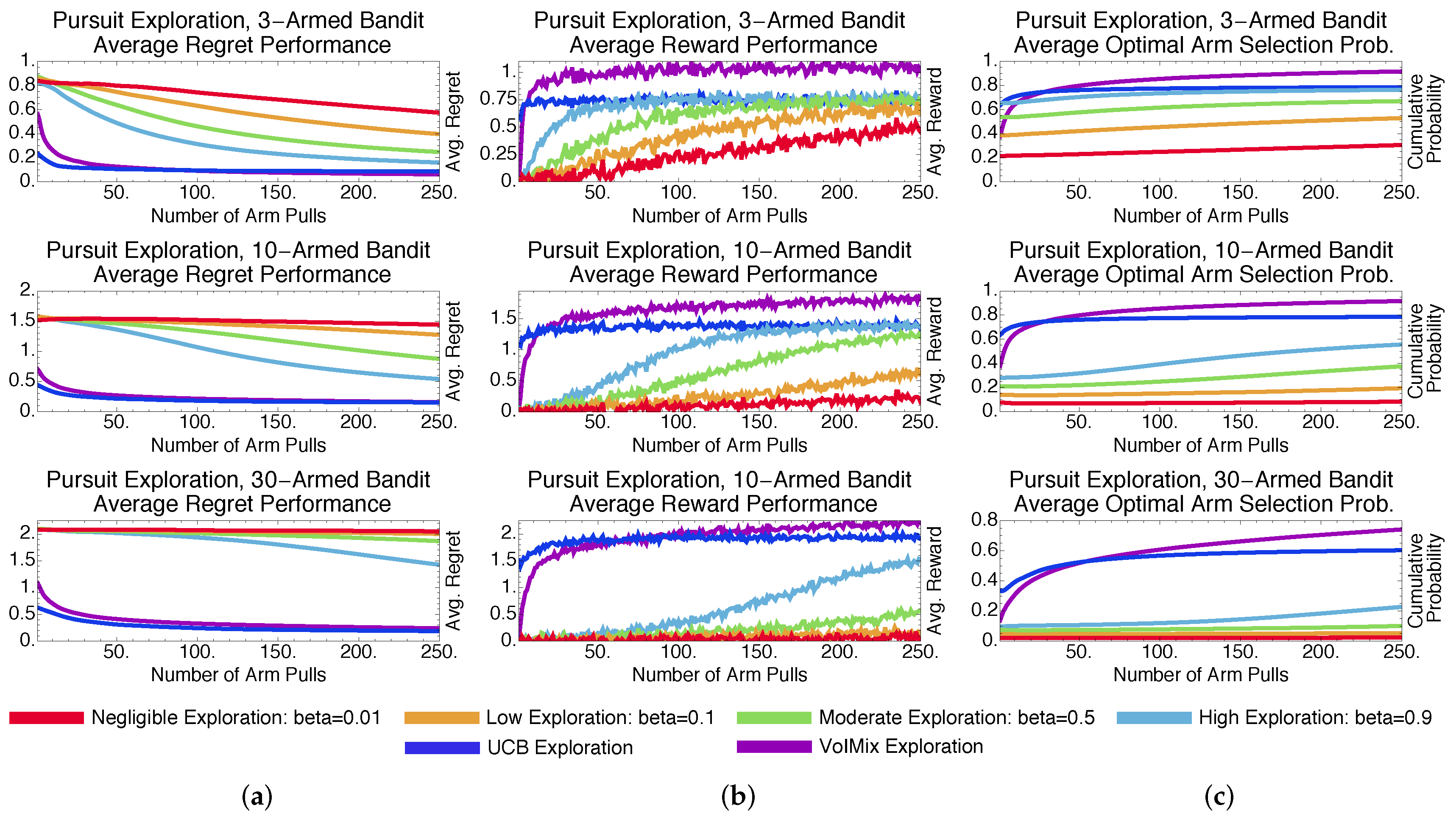
I have read Sutton's book and an tutorial regarding this learning. The duration is 10. am learning about reinforcement learning and came across the first and simplest form of reinforcement learning system called multi-armed reinforcement learning (also called as n-armed bandit). This project receives funding from the German Ministry of Education and Research (BMBF) as a part of the program “promoting young female scientists in artificial intelligence”. The application area of the results goes beyond wireless communications, ranging from science to engineering to digital health and digital humanity. Moreover, based on the analytical and numerical results, MABISS plans to develop an intelligent spectrum sharing testbed. Taking the physical characteristics of wireless networks into account, MABISS investigates the problem by practicing the theory of multi-agent multi-armed bandit and providing performance bounds. Motivated with the ever-increasing demand for wireless spectrum, the application-wise focus of MABISS is the distributed intelligent spectrum sharing challenge for device-to-device communications, which is a key enabler of the emerging networking paradigms such as the Internet of Things, edge/fog computing, and small cell networks. These include fully-distributed bandit games, bandit mechanism design, network bandits, and human bandits. With a forward-looking vision, the project MABISS aims at developing rigorous theoretical frameworks to address the multi-agent multi-armed bandit problem in different settings, particularly those that frequently arise in real-world applications. Such scenario, which is located at the intersection of two pillars of artificial intelligence, namely, decision-making under uncertainty and multi-agent systems, requires analysis not only based on the regret performance, but also involving the concepts such as equilibrium, fairness, incentive-compatibility, revenue, and diffusion. While the seminal formulation comprises only one player that faces the exploration-exploitation dilemma, the challenge becomes significantly aggravated in the multi-agent setting, where the decision-makers mutually affect each other while sharing limited resources. Multi-armed bandit is a variety of sequential decision-making problems under uncertainty, envisaged by a gambler playing on a slot machine.
